Strategy
OPINION OF THE WEEK: Artificial Intelligence – Racing Up The Mountain Without A Harness
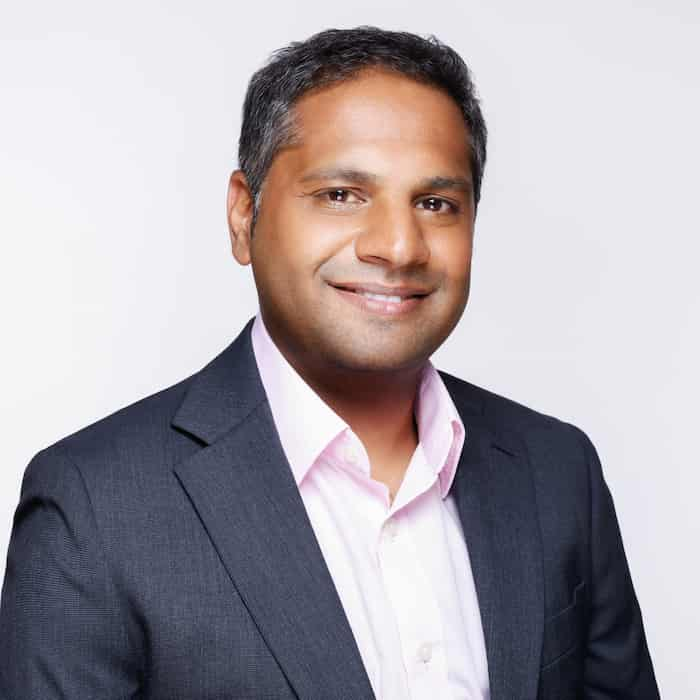
Ensuring the safe and controlled use of AI systems is crucial, the author of this article writes.
The editor of this publication is taking a break from weekly commentaries to give the floor to an outside contributor. The following article looks at how firms which adopt AI technology could set themselves up for legal trouble. The piece comes from Akber Datoo at D2 Legal Technology. It examines the commercial and regulatory implications of unmanaged and the immature use of AI. The editors know that AI continues to be a major topic for global wealth management in all its forms. This article is an important contribution to this discussion. The usual editorial disclaimers apply to views of guest writers. Please get involved in the conversation! Email tom.burroughes@wealthbriefing.com
The speed with which businesses globally have adopted generative
AI tools over the past 12 months has been extraordinary. It took
Netflix three and a half years to achieve one million customers
and Twitter two years, while Instagram achieved that number in
just two and a half months. ChatGPT hit the million-user mark in
just five days. The business implications are significant and
cannot be ignored. According to the latest annual McKinsey
Global Survey, while one-third of organisations are already
using generative AI in at least one business function, only a few
of them have put in place robust AI usage frameworks.
While many are concerned about the impact of AI on white collar
jobs, CEO Akber Datoo and consultant Jake Pope at D2 Legal
Technology, argue that financial institutions should have far
bigger concerns about the commercial and regulatory implications
of unmanaged and immature use of AI on internal
processes.
Concerns should include the state of existing data – in terms of
the inputs into an AI system – data governance in downstream
systems, and the training set data used in Large Language Models
(LLMs). There is an urgent need to assess and mitigate possible
risks and create robust policies for the managed adoption of AI
across an organisation.
Misplaced fears
The global financial market is at the vanguard of AI adoption.
The 60 largest North American and European banks now employ
around 46,000 people in AI development, data engineering and
governance and ethics roles, with as many as 100,000 global
banking roles involved in bringing AI to market. Some 40 per
cent of AI staff in these banks have started their current
roles since January 2022, underlining the speed with which
organisations are ramping up their AI adoption. Meanwhile
the UK fears that its banks could be falling behind their US
counterparts, with American giant JP Morgan hiring twice as many
AI-related jobs as any of its rivals.
This AI hiring fiesta is causing serious concern amongst existing
employees, with many worrying that they will be displaced. How
long, they wonder, will it take a generative AI tool to learn the
skills and knowledge individuals have taken years to attain?
Indeed, those working in the technology and financial-services
industries are the most likely to expect disruptive change from
generative AI. Fears have been further fuelled by organisations
such as the World Economic Forum which claims that 44 per cent of
workers' core skills are expected to change in the next five
years.
But such fears fundamentally overlook the far more significant
concerns regarding the way organisations, especially those within
banking, are approaching AI adoption: far too few are actively
considering, in contrast to the promise of these tools, the
significant business risks. Generative AI is still in a very
immature phase. If organisations remain bedazzled by the possible
efficiency and cost savings on offer and fail (through lack of
policies, procedures and training) to consider the risks of
discrimination, bias, privacy, confidentiality and the need to
adhere to professional standards, the outcome could be
devastating.
Lack of strategic oversight
Organisations are not taking the time to consider AI usage
policies. They are not drawing clear distinctions between the
personal and professional use of AI. Indeed, due to the
difficulty in identifying where and when AI has been used, many
companies are blind to how, when and where AI is being used
throughout the business. According to McKinsey, just 21 per cent
of respondents reporting AI adoption say their organisations have
established policies governing employees’ use of generative AI
technologies in their work.
These are concerning issues in any business, but within the
highly regulated financial sector, the level of risk being
incurred is jaw dropping. Taking the derivatives world as an
example, some firms have already mooted the use of AI to
streamline close out netting for their derivatives contracts yet
often the quality of data held within financial institutions is
fundamentally inadequate. What will happen if organisations start
training generative AI tools on inaccurate data, as a supposed
efficiency, while the human skillset to review and use data
responsibly is gradually being lost?
We regularly hear of the desire to scrap (often offshored)
data extraction exercises from large portfolios of ISDAs, GMRAs,
GMSLAs, MRAs, MSFTAs etc. given the challenges legal agreement
data and trade linkage continues to cause for resource (across
for example capital, liquidity and collateral) optimisation,
regulatory compliance/reporting and operational management.
It is easy to dream of the magic AI bullet, yet a deeper look
will show that this is, in fact, a data nightmare. Any data
scientist will tell you of the magic mantra “garbage in means
garbage out.”
AI usage policies and frameworks, dovetailing with mature data
governance, are critical to ensure that firms do not run blindly
into costly AI projects that are doomed to fail.
Unknown risks
Of course, organisations recognise that there is a problem
with the lack of accurate, trusted data required to train
newfangled AI tools. But turning instead to synthetic data
sources is not a viable solution. Worryingly, there are several
requests being seen from organisations to create synthetic
documents in order to sufficiently “train the AI” and meet
minimum training set numbers given to them by AI vendors, and
therefore exacerbating the issues of hallucinations, bias and
discrimination.
Not only is the current data resource inadequate, but the
immaturity of AI will continue to create unacceptable risk.
Drift, for example, is a significant concern. In machine
learning, “drift” refers to when LLMs behave in unexpected ways
that move away from the original parameters. Carefully defined
workflows can then suddenly behave unexpectedly and cause
significant issues downstream.
One thing is very clear is that the pivotal role of the
“human-in-the-loop” in any use of AI is something that needs
to be central to AI usage policies.
Financial regulators are likely to take punitive action against
any organisation opting to fast-track compliance through the use
of AI without the right controls in place. Even if AI legislation
is still in its infancy, there are still risks of breaching
existing laws pertaining to discrimination and competition.
There are also emerging AI-specific regulatory concerns,
especially within the EU. The draft negotiation mandate for the
EU AI Act, recently endorsed by the European parliament, has been
heralded as European lawmakers setting the way for the rest of
the work on “responsible AI.” The new act targets high-risk
use cases rather than entire sectors, and proposes penalties of 7
per cent of turnover or €40 million ($42.4 million) – in excess
of existing GDPR fines.
Evolving risk perception
While market participants debate the best way to proceed,
organisations need to consider the implications of their current
laissez-faire approach to AI exploration. The EU has taken a very
different stance to the US and UK, compounding the difficulty for
even those who seek to embrace AI carefully.
The incident with Samsung employees loading confidential client
data into a generative AI tool highlights the implications of the
lack of guidelines and training for usage. The security
implications associated with hallucinations, jailbreaking or
smart prompting are clear, and there have been incidents that
have prompted several high-profile organisations to ban the use
of generative AI at work.
There are also huge class action lawsuits under way against
companies such as Open AI about the use of personally
identifiable data and whether it goes beyond the principle of
fair use.
Why are so many firms failing to balance positive AI innovation
with managing the risks? The answer is likely that this is
untested ground and, without regulation, it is all too easy
to gallop ahead. AI systems must be constantly and periodically
monitored, reviewed, and audited. Firms need to create robust AI
usage policies and undertake continual assessment of the
potential impact on existing policies, from cybersecurity to data
protection and employment.
Conclusion
The current attitude of companies and financial institutions to
the adoption and use of generative AI is astonishing. How can
global financial banks, organisations that are still enduring the
fall-out of the Lehman’s failure in 2007, embark on such
speculative activity without recognising the extraordinary risk
implications? Now is the time for commercial responsibility, wise
management oversight, and risk weighted judgement.
Ensuring the safe and controlled use of AI systems is crucial.
Contrary to many statements, it is easy to write regulation for
AI. It is hard to ensure systems comply. This is why the way we
use AI is critical.
About the author:
Akber Datoo, founder and CEO of D2 Legal Technology (D2LT), is also a professor at the University of Surrey; a technologist (ex-UBS front office fixed income derivatives IT) and a derivatives lawyer (ex-Allen & Overy). He has been appointed to the Technology and Law Committee of the Law Society of England and Wales in 2016, with a specialism in Big Data, artificial i ntelligence, distributed ledger technology, and smart contracts.